Developed SinkPIT, a sound source separation technology for environments with many speakers speaking at the same time
- Voice and Acoustic Processing
Overview
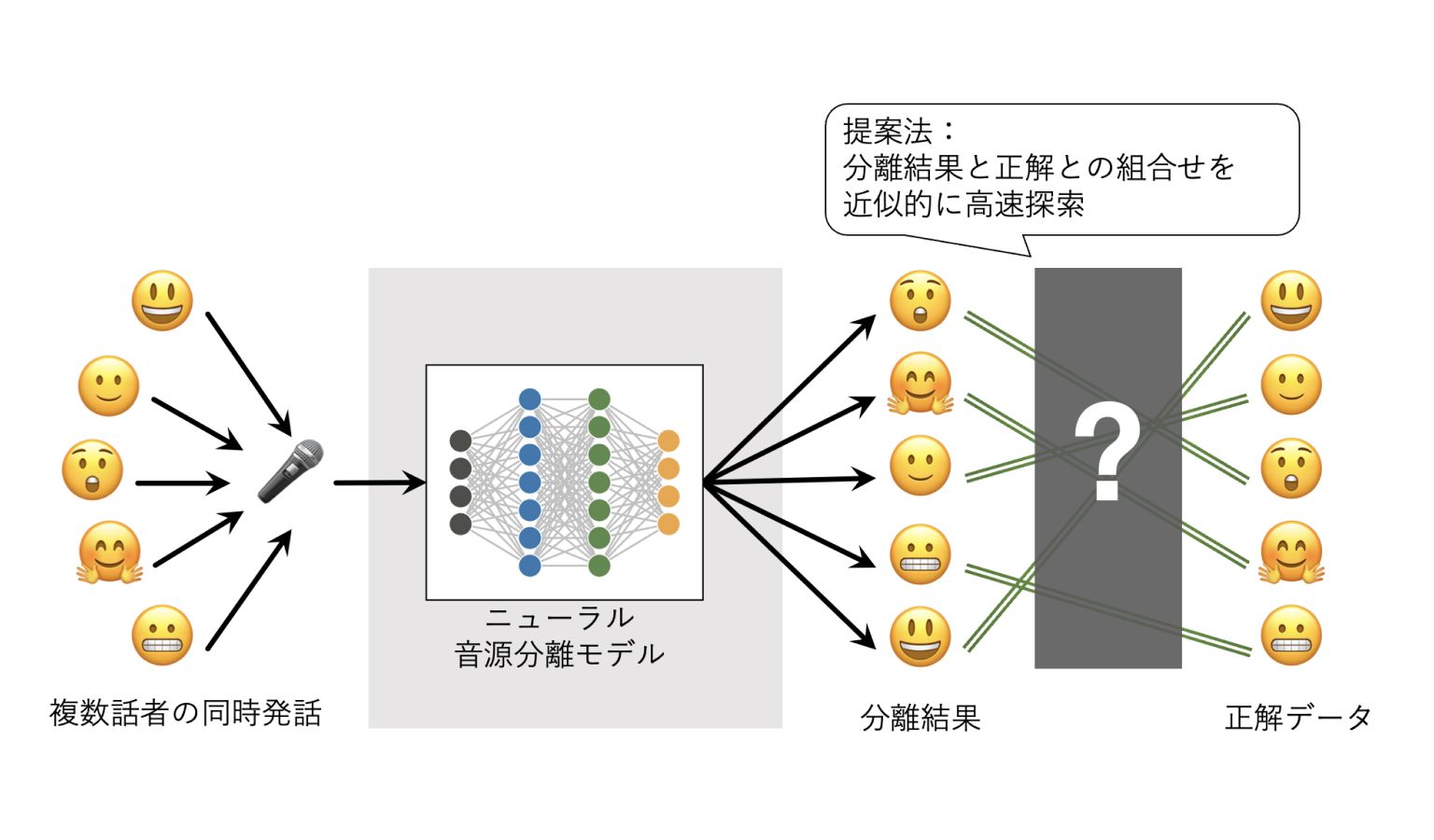
- Project period
- October 7, 2020 (Publication of Accepted Paper Information)
PKSHA Technology Inc. has developed "SinkPIT", a deep learning technology for separating speech for each speaker in an environment where many speakers are speaking simultaneously.
"SinkPIT" has been experimentally demonstrated to be capable of learning a large-scale sound source separation model with a larger number of assumed sound sources than before in a realistic computational time. By combining this technology with various speech signal processing technologies, we expect that it can be applied to various applications, such as conference proceedings systems and in-vehicle microphones.
Technology
In recent years, spoken dialogue systems, including in-store customer service robots and smart speakers, have become increasingly popular. The technology of "sound source separation" has long been widely studied and utilized as an important elemental technology for such spoken dialogue systems. This is a pre-processing technique that separates speech into individual speakers in situations where multiple speakers are speaking at the same time. It is believed that sound source separation can improve speech recognition performance in situations where multiple speakers are speaking at the same time.
In recent years, with the development of deep sound source separation based on deep learning, it has become possible to separate the sound sources of two to five speakers with a fairly high sound quality. However, these methods are computationally expensive in the learning process (brute force search), so it is difficult to increase the number of assumed sound sources (the maximum number of speakers to be separated).
In light of this background, PKSHA Technology has developed SinkPIT, a learning method for deep sound source separation that makes it possible to significantly increase the number of assumed sound sources for deep sound source separation. Experimental results demonstrated that SinkPIT can train a large-scale sound source separation model with a larger number of assumed sound sources in a realistic computational time. This technology is expected to be applicable to various applications such as conference proceedings systems and in-vehicle microphones by combining it with various speech signal processing technologies.
The results were presented at the IEEE ICASSP (International Conference on Acoustics, Speech and Signal Processing) 2021 held in Toronto, Canada in June 2021. (Paper presented and published online.)
Member in charge
TACHIBANA hideyuki
Graduated from the Department of Mathematical Engineering, Faculty of Engineering, The University of Tokyo. D. from the Graduate School of Information Science and Engineering, The University of Tokyo.
D. in Information Science and Engineering.
After working as a researcher at Meiji University, joined PKSHA Technology.
Engaged mainly in research and development of speech processing, language processing, and signal processing.KATAYAMA yotaro
He holds a Bachelor of Engineering degree from the University of Tokyo.
After working for a foreign investment bank, he joined PKSHA Technology.
Served as a director of BEDORE Co.
He is currently a director of MNTSQ Corporation.INAHARA munehiro
Graduated from the Department of Electronics and Computer Engineering, Faculty of Engineering, The University of Tokyo.
During his studies, he researched game AI and natural language processing technology.
After graduation, he joined IBM Japan.
At Tokyo Systems Development Laboratory, he was in charge of research and development of systems and solution business mainly based on Watson and deep learning.
After joining PKSHA Technology, he has been engaged in research and development of many products and modules such as spoken dialogue products, cause-and-effect recognition, emotion recognition, language models, and speech synthesis.